Собрал LSTM Multiple Input Multi-Step Output из библиотек TF keras обучил на датасете из 4000 по каждому столбцу, столбцов 10. до 3800*10 на обучение 100*10 на тест и остальные 100*10 на сверку с прогнозом. Получил какую то чушь. Эпох 50. лернинг рейт 0.015.
Так выглядят данные
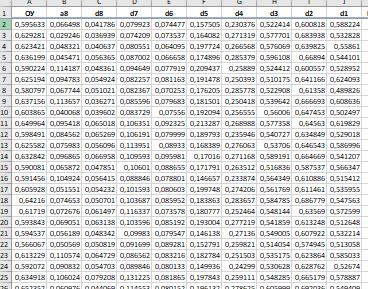
Первый график прогноз
(сверочный
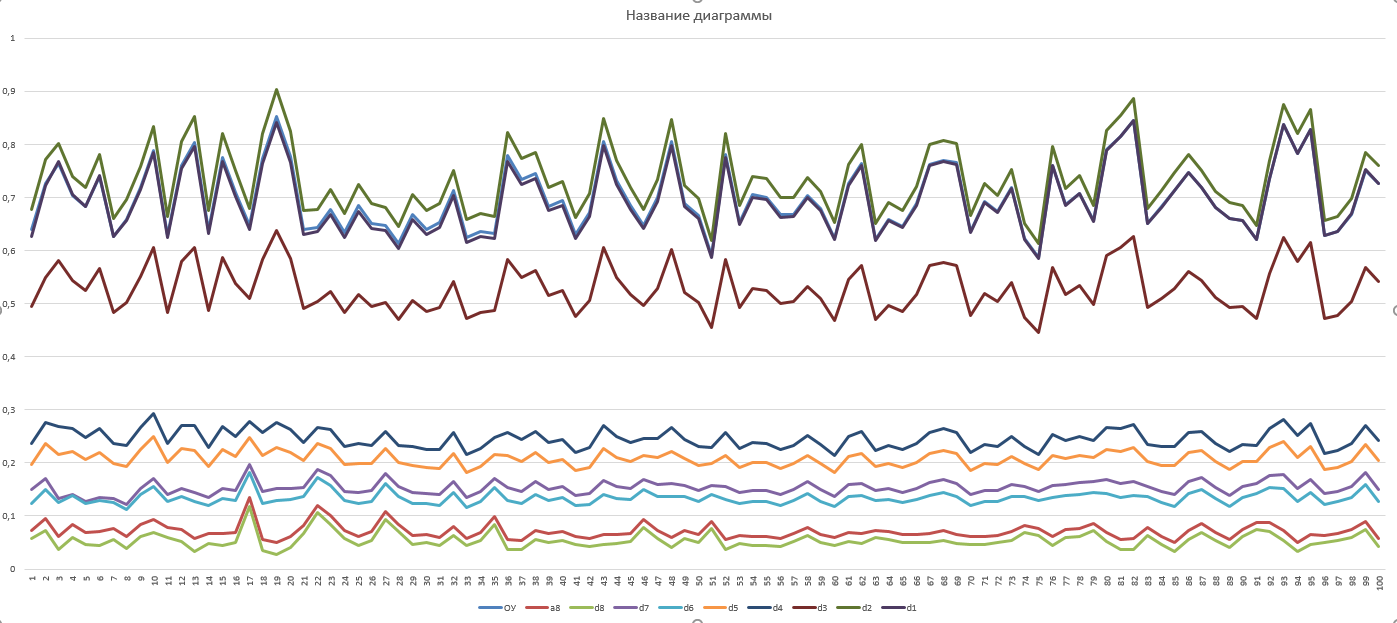
)
Второй оригинальный
(сверочный

)
class _LSTM_():
n_steps_in = None
n_features = None
n_steps_out = None
model = None
def __init__(self, n_steps_in, n_steps_out, n_features):
self.n_steps_in = n_steps_in
self.n_steps_out = n_steps_out
self.n_features = n_features + 1
pass
def init_parameters(self, n_layer1=300, n_layer2=300):
self.model = Sequential()
self.model.add(LSTM(n_layer1, activation='tanh', input_shape=(self.n_steps_in, self.n_features)))
self.model.add(RepeatVector(self.n_steps_out))
self.model.add(LSTM(n_layer2, activation='tanh', return_sequences=True))
self.model.add(TimeDistributed(Dense(self.n_features)))
# clipvalue
# Gradient value clipping involves clipping the derivatives of the loss function to have a given
# value if a gradient value is less than a negative threshold or more than the positive threshold.
# For example, we could specify a norm of 0.5, meaning that if a gradient value was less than -0.5,
# it is set to -0.5 and if it is more than 0.5, then it will be set to 0.5.
self.model.compile(optimizer=optimizers.Adam(learning_rate=0.015), loss='mean_squared_error')
def __split_sequences(self, _sequences, n_steps_in, n_steps_out):
X, y = list(), list()
for i in range(len(_sequences)):
# find the end of this pattern
end_ix = i + n_steps_in
out_end_ix = end_ix + n_steps_out
# check if we are beyond the dataset
if out_end_ix > len(_sequences):
break
# gather input and output parts of the pattern
seq_x, seq_y = _sequences[i:end_ix, :], _sequences[end_ix:out_end_ix, :]
X.append(seq_x)
y.append(seq_y)
return array(X), array(y)
# [ [,,,],
# [,,,],
# [,,,],
# [,,,]]
def train(self, x: array, eps=200, _vse=0):
countRow = x.shape[0]
countItems = x.shape[1]
summ = 0
out_seq = []
for col in range(countItems):
for row in range(countRow):
summ += x[row][col]
out_seq.append(summ)
summ = 0
out_seq = array(out_seq)
x = transpose(x)
out_seq = out_seq.reshape((countItems, 1))
dataset = hstack((x, out_seq))
X, y = self.__split_sequences(dataset, self.n_steps_in, self.n_steps_out)
self.model.fit(X, y, epochs=eps, verbose=_vse)
pass
def save(self, path: str):
self.model.save_weights(path)
# [[,,,],[,,,],[,,,],[,,,]]
def predict(self, x: array, _vse=0):
countRow = x.shape[0]
countItems = x.shape[1]
summ = 0
out_seq = []
for col in range(countItems):
for row in range(countRow):
summ += x[row][col]
out_seq.append(summ)
summ = 0
out_seq = array(out_seq)
x = transpose(x)
out_seq = out_seq.reshape((countItems, 1))
x_input = hstack((x, out_seq))
# [[1, 4, 5], [2, 5, 7], [3, 6, 9]]
x_input = x_input.reshape((1, self.n_steps_in, self.n_features))
return self.model.predict(x_input, verbose=_vse)
def loadData(name, start, end):
pdf = pd.read_excel(open('C:\\Users\\Pantuchi\\Desktop\\Данные Александру.xlsx', 'rb'), sheet_name=name)
s = []
s.append(pdf['ОУ'][start:end])
s.append(pdf['a8'][start:end])
s.append(pdf['d8'][start:end])
s.append(pdf['d7'][start:end])
s.append(pdf['d6'][start:end])
s.append(pdf['d5'][start:end])
s.append(pdf['d4'][start:end])
s.append(pdf['d3'][start:end])
s.append(pdf['d2'][start:end])
s.append(pdf['d1'][start:end])
s = array(s, dtype=float)
return s
if __name__ == '__main__':
a = loadData('ВУ_Неисправный', start=0, end=3800)
scaler = StandardScaler()
scaler.fit(a)
a = scaler.transform(a)
l = _LSTM_(100, 100, a.shape[0])
l.init_parameters()
l.train(a, eps=200, _vse=1)
l.save('D:\\Aleksandr\\Documents\\PycharmProjects\\LTSM1\\checkpoint')
b = loadData('ВУ_Неисправный', start=3800, end=3900) # 100 для сверки
scaler.fit(b) # shape(10, 100)
a = scaler.transform(b)
p = l.predict(a) # shape(1, 100, 11)
p = transpose(p) # shape(11, 100, 1)
p = resize(p, (10, 100)) # shape(10, 100)
p = scaler.inverse_transform(p)
p = transpose(p) # shape(100, 10)
pdf = pd.DataFrame(p, columns=['ОУ', 'a8', 'd8', 'd7', 'd6', 'd5', 'd4', 'd3', 'd2', 'd1'])
pdf.to_csv('predict1.xlsx')
print(pdf)
P.S. Буду очень признателен за подсказки в каком направлении копать